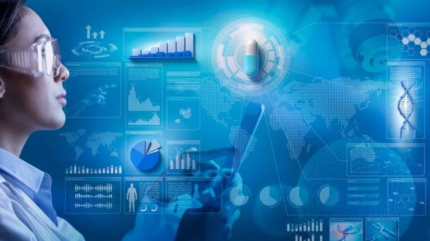
With 2025 well underway, the runaway momentum of artificial intelligence (AI) looks unstoppable. GlobalData estimates that the total AI market will be worth over $1 trillion by 2030. As with many sectors, this rapid growth is already having a transformative impact in life sciences.
According to GlobalData’s Drugs database, there are over 3,000 drugs that have been developed or repurposed using AI as of the beginning of 2025. The majority are in early stages of development — either discovery or preclinical. Oncology is the leading therapy area, followed by the central nervous system, infectious disease and immunology. These numbers are only set to grow.
Beneath the eye-popping headlines, advances being made in some of the most technically demanding areas of pharmaceutical development — like manufacturing proteins with unprecedented binding affinities and generalizability — is astounding. Getting to grips with these changes, and how AI is fueling them, sheds light on how the whole world of life sciences is being turned upside-down by the technology’s tremendous potential.
AI and protein design
Proteins are the fundamental building blocks of biological function. As firms involved in their design know, making new proteins capable of specific, high-affinity interactions is no easy task. Historically, engineered proteins have been derived from native proteins through approaches like directed evolution and immunization. While effective, these methods offer limited control over epitope targeting and require extensive laboratory screening. Computational de novo design, in which proteins are designed “from scratch” based on physical principles, is a powerful tool. But it may also necessitate multiple rounds of experimental optimization to achieve high-affinity interactions.
AI-driven protein design is revolutionizing the field of biotechnology, enabling the creation of proteins with unprecedented precision and efficiency. Leveraging advanced machine learning models, these systems are transforming how researchers approach protein engineering. Generative AI models are trained on vast datasets of structural and sequence information, allowing them to design proteins that interact specifically with predefined molecular targets. By optimizing binding affinities and minimizing the need for extensive experimental screening, these innovations can greatly improve on traditional computational and experimental methods. For example, researchers in 2024 reported a system capable of generating designs that exhibit binding affinities in the picomolar to low-nanomolar range, outperforming traditional methods by factors ranging from 3- to 300-fold across multiple targets.[1] The resulting proteins showed that this technology has immense potential in therapeutic research and development — from tackling SARS-CoV-2 to advancing cancer and autoimmune disease treatments.
What sets AI systems apart is their ability to create structurally diverse, stable and highly functional protein binders in a streamlined and scalable manner. Through AI-driven scoring mechanisms and iterative refinement, researchers can prioritize high-confidence candidates for validation, accelerating discovery pipelines. Researchers using AI have successfully designed binders for critical therapeutic targets like VEGF-A and IL-7RA, where conventional methods have faltered.1 As these platforms continue to evolve, they hold great potential for addressing complex biological challenges and unlocking new possibilities in medicine and biotechnology.
Confronting challenges
Despite this remarkable progress, AI-powered drug design is still in its infancy, and is not a panacea. Certain protein targets, such as TNF-α, have so far eluded computationally designed binders. Furthermore, molecules representing lead candidates for clinical evaluation may require multi-parametric optimization of unpredictable pharmacologic and pharmacodynamic characteristics like in vivo half-life, tissue distribution and off-target side effects.
Meanwhile, there are other problems endemic to the pharmaceutical world that AI still struggles to tackle. For example, one meta-study of 1,300 papers found that 10% contained manipulated images. With blot imaging so susceptible to manipulation, yet still widely accepted as proof for peer review purposes, scientific publishers are investing heavily in AI tools to weed out manipulation.[2] But even innocuous edits — such as adjusting image intensity — can trigger rejections, leading to potentially costly hold-ups.
As additional computational advancements trickle into pharmaceutical development — like integration of AI with quantum computing, and ever-enhancing training datasets — these are the kinds of shortcomings researchers hope to tackle. And new solutions are entering the market all the time to tweak the efficiency of AI tools. Publishers’ quandaries around blot imaging provides an instructive example. Cytiva’s free image integrity checker, for example, generates a unique, unalterable hash-tagged fingerprint for raw images. Any modification alters this tag, ensuring traceability and allowing researchers to verify up to 1,000 images in seconds.[3] By streamlining verification and maintaining data authenticity, the tool helps labs uphold high scientific standards while reducing delays and safeguarding research integrity.
It is clear that AI will enable drug developers to expedite their fight against diseases. While monoclonal antibodies, for instance, are a mature technology with wide therapeutic relevance, they are relatively labor-intensive and costly to optimize and produce. AI-designed binders can be made smaller and more thermostable, and they are easier to manufacture — making them a valuable and game-changing addition to the biotherapeutic toolbox.
Trailblazers leading the way
What are some of the major frontiers of AI-driven drug design? Wide areas are ripe for enhancement, as examples from the industry’s top innovators reflect. One of these is affinity chromatography, a cornerstone of protein purification. Affinity chromatography enables the selective separation of biomolecules through specific interactions between a target protein and a complementary ligand. Traditionally, identifying these ligands has been a labor-intensive process. But today it is a key area where AI is promising change. Cytiva is one company at the forefront of doing just that, harnessing AI to accelerate ligand discovery, optimize binding specificity and enhance manufacturability.
Cytiva’s approach involves exhaustive phage display screening, where researchers sift through hundreds of billions of sequences to identify candidates with the right affinity, specificity and stability. AI offers a game-changing approach to catalyzing this process, predicting ligand sequences computationally and significantly reducing the experimental burden — greatly accelerating discovery timelines.
“We’ve been embarking on this journey, it’s early days, but it’s exciting, we’ve been putting in place an artificial intelligence tool at Cytiva that is able to predict such new ligand sequences,” says Dr. Beate Mueller-Tiemann, Chief Technology Officer at Cytiva. “The first couple of thousands of predicted sequences have been generated and are now in confirmatory experiments.” Cytiva’s AI platform has proven its predictive power in generating sequences with optimal binding characteristics to generate novel ligand sequences. With this capability undergoing validation, exciting milestones in the field of bioseparation lie ahead.
Another example is use of machine learning to analyze early drug candidates. Surface plasmon resonance (SPR) is a powerful, real-time analytical technique that detects and characterizes molecular interactions, providing critical insights into binding affinity and kinetics— key factors in drug discovery. Recent advancements in AI are revolutionizing how SPR data is processed, tackling issues around inefficiency, variability and data bottlenecks. A newly developed machine learning solution for SPR data analysis, also from Cytiva, has shown significant promise, reducing analysis time by up to 90%. The prototype also corroborated research by Biacore™ experts in 95% of cases, highlighting its precision. By automating laborious manual steps, this approach enhances both productivity and data reliability, enabling scientists to focus on decision-making and advance candidates more effectively.
Considering that only about 10% of drugs in Phase I trials are likely to reach FDA approval, such innovations offer a streamlined pathway to developing novel therapeutics. The solution’s transparent and trainable design allows researchers to refine the model for their specific needs while benefiting from pre-trained workflows that minimize onboarding time. By making SPR analysis faster and more consistent, it means the full potential of this information-rich technique can be realized in drug discovery — critically, in the early stages of identifying and optimizing candidates.[4]
According to Dr. Mueller-Tiemann, this is just the beginning: “We will evolve our capabilities and the tools to… [accelerate] the discovery of new affinity chromatography ligands and solutions for our customers to accelerate the discovery and the manufacturing of innovative biotherapeutics,” she says. Similar genAI principles could be extended to enable the design of new chromatography base matrices with enhanced performance characteristics. This application of AI holds the potential to redefine the biopharmaceutical purification landscape, supporting the discovery and manufacturing of next-generation biologics.
As AI-powered tools continue to evolve, Cytiva’s computational models will advance and become more refined alongside them. By leveraging AI to optimize affinity chromatography technologies, Cytiva is leading the quest for faster, more efficient purification solutions, ultimately enabling scientists to accelerate the development of innovative biotherapeutics and life-saving treatments. Are you prepared for an AI-driven future? To learn more about the future of life sciences, the importance of AI in driving next-generation protein designs and how working with Cytiva can keep your life sciences firm abreast of change, fill in your details to download the report on this page.
[1] Zambaldi, Vinicius et al. “De novo design of high-affinity protein binders with AlphaProteo.” arXiv preprint arXiv:2409.08022 (2024).
[2] Bucci, E.M. Automatic detection of image manipulations in the biomedical literature. Cell Death Dis 9, 400 (2018). https://doi.org/10.1038/s41419-018-0430-3
[3] https://www.cytivalifesciences.com/news-center/image-integrity-in-scientific-research-10001
[4] https://www.cytivalifesciences.com/solutions/protein-research/interaction-analysis-with-biacore-surface-plasmon-resonance-spr/key-spr-applications/small-molecule-drug-discovery/transparent-machine-learning