Artificial intelligence (AI) has the potential to revolutionize drug development through improved efficiency, accuracy, and speed. Research indicates biopharma companies have been slow in applying AI and digital technologies compared to other industries. CROs/CDMOs can act as a bridge between biopharma companies and evolving technologies such as AI across the biopharma value chain.
This article explores key considerations when using AI and how leading CRO/CDMO Syngene uses AI and digital technologies to accelerate drug discovery, development, and manufacturing for its clients.
Critical questions for applying AI and digital technologies
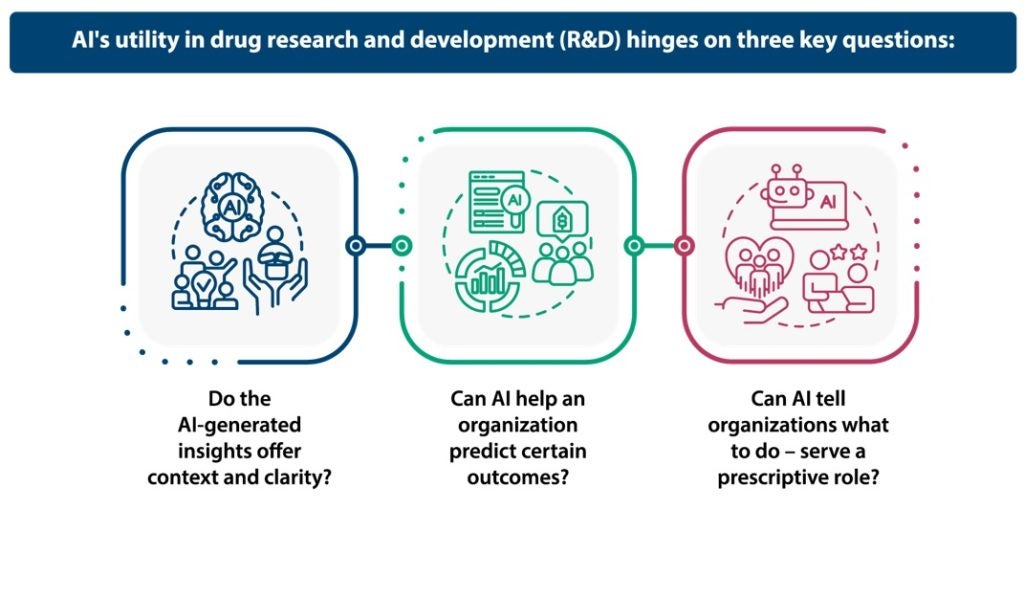
AI-driven decisions during drug discovery, and R&D in general, are critical, given that the industrywide success rate of drug development processes currently hovers between 10% and 15%1.
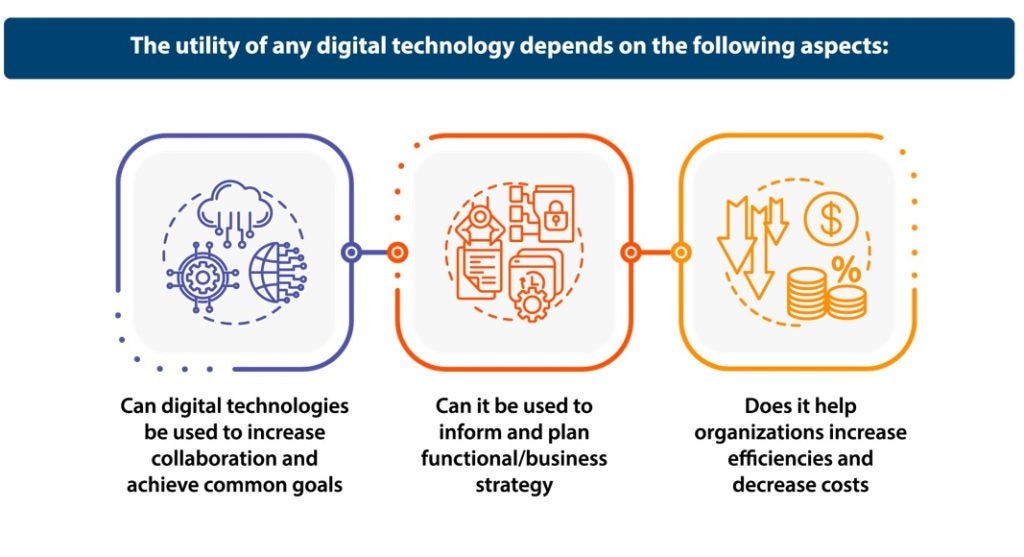
AI application areas in biopharma
Target Identification and Drug Discovery
AI algorithms analyze vast amounts of biological data, including genomic, proteomic, and metabolomic data, to delineate pathways associated with disease and identify potential drug targets.
Similarly, learning models predict the affinity of small and large molecules to target proteins, facilitating the virtual screening of compounds. Predictive models can predict drug-like properties and liabilities of molecules, thereby helping in the design and prioritization of lead candidates with the desired target product profile.
This action is carried out in most biopharma companies today, with the industry working toward more useful models to predict biological properties. AI thus minimizes late-stage attrition and increases compliance and chances of commercial success. The application of AI can also significantly reduce the DMTA cycles, saving time and money.
According to a report released in June 2023 and co-authored by BCG and the research funder Wellcome, AI could yield "time and cost savings of at least 25–50%"2 in drug discovery up to the preclinical stage.
Drug Repurposing
AI analyzes large-scale biological and clinical datasets to identify existing drugs that may be repurposed for new indications. Deep learning algorithms predict the therapeutic potential of known drugs based on their molecular profiles and biological activity, thereby accelerating the identification of new treatment options for various diseases, especially rare and neglected diseases.
Biomarker Discovery and Patient Stratification
AI analyzes omics data to identify biomarkers associated with disease progression, treatment response, and patient outcomes. Algorithms can stratify patient populations based on molecular signatures, enabling personalized treatment approaches and improving the efficacy of therapies.
Development and manufacturing processes
Quality is of paramount importance in the development of biopharmaceutical manufacturing processes. Quality stands to benefit significantly from the application of AI and digitization, as the integration of process analytical technologies (PAT), sensors, and multivariate data analytics create a knowledge-driven quality-by-design (QbD) approach. This approach allows for the efficient management of areas of variability crucial for the success of patient-specific therapies. This integration, along with data records and enterprise systems, further facilitates seamless knowledge-sharing during the development of manufacturing processes and controls.
In manufacturing, AI currently serves three primary application areas. First is the correlative/descriptive role, which comprises producing batches or conducting small experiments to design each batch and then finding correlations between various parameters that allow scientists to create optimal parameter setups.
The second role is predictive, where AI analyzes breakdowns in machinery, fault tolerance, batch tolerance, purity profile, and more. For example, how often is a batch failing, and the reason for those failures? From data generated by the manufacturing process, AI can build models, pinpoint timestamps or production steps where machinery is failing, and then suggest a maintenance schedule to help fix the problem. This role is common across different industries.
The third application area relevant to manufacturing involves using AI to streamline manufacturing. For example, what are the inputs for the raw material, and what scale can generate APIs of the right purity? AI can also guide researchers toward the optimal methodology for that particular API and a more efficient process model for maximum output.
References