
When artificial intelligence (AI) hits the headlines, it’s usually bad news pertaining to the perils of face recognition. It was only recently that Twitter had to remove an AI-based cropping tool due to its bias against images of black people; more often than not, only lighter skin tones would be picked up by the computer vision employed.
Google’s own visual service used to label images of dark-skinned individuals holding a thermometer as in fact containing a gun, doing no such thing for light-skinned subjects. In 2018 meanwhile it was revealed that facial recognition systems developed by Microsoft, IBM, and Megvii had an error range of 35% when determining the gender of dark-skinned women, compared with less than 1% for light-skinned men.
These flaws in AI training give the technology a bad name, and so do regular media reports suggesting that intelligent machines are poised to decimate the human workforce. These themes, for many people, have obscured AI’s genuine usefulness in data analysis and conversational platforms. And while computer vision does indeed have its flaws, it is more than just a reflection of societal biases: it is potentially an essential tool for both society and business.
Beyond face recognition: What exactly is computer vision?
Computer vision, or CV, gives machines the power of visual recognition in a way that emulates human sight. Whether a machine is detecting dangers on the road or, more controversially, recognising faces in a crowd, the ultimate aim is to make decisions based on image interpretation.
The tech is an advanced form of pattern recognition, made through statistical comparison of data sets. This means that while machines can “see”, they have no real understanding of what they are looking at. They can distinguish one object from another, true, but can’t explain what this difference means.
“As the system doesn’t know what a cat is, it would almost certainly fail to recognise a real cat outside of the confines of a still image,” as a GlobalData thematic report on computer vision explains.
How well do you really know your competitors?
Access the most comprehensive Company Profiles on the market, powered by GlobalData. Save hours of research. Gain competitive edge.
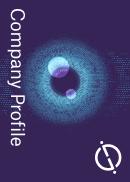
Thank you!
Your download email will arrive shortly
Not ready to buy yet? Download a free sample
We are confident about the unique quality of our Company Profiles. However, we want you to make the most beneficial decision for your business, so we offer a free sample that you can download by submitting the below form
By GlobalDataTo classify digital images, computer vision tech uses image recognition algorithms that are trained to identify differences in digital images of different classes. To this end, an AI system is shown thousands of images, some of which contain the object or class of objects the algorithm is being trained to identify (for instance, a cat) and some of which don’t. In order for the AI to learn, the images need to be labelled (in this example, the pictures need to be labelled as “cat” or “no cat”), so that the system can tell when it is getting the task right. The more images it processes, the better the algorithm becomes at classifying them. But if the quality of the training data is flawed (for instance if only ginger cats are pictured) this compromises the machine’s output (it will probably fail to identify non-ginger cats as being cats) – and such flawed training data has led to the various Big Tech blunders of bias that keep appearing in the news.
The Four Eyes of AI
Image recognition is one of four technology categories which make up CV, alongside object recognition, video recognition and machine vision (MV). Object recognition follows the same process of image recognition in that it also assigns a class label to which the viewed image belongs. However it is also able to locate the object in the image, drawing a bounding box around it.
Video recognition software meanwhile analyses video clips, compares them to a database of content and determines if there is a match.
The outlier is machine vision, a hybrid solution of software and hardware. This tech can both inspect for, say, missing and defective parts, and guide robots through visual feedback as they move around the factory floor. As an example, a smart camera can be programmed to detect component flaws and, if networked to something like a robotic arm or retractor, signal for the removal of a defective product on the assembly line.
Business vision
It may not be surprising to learn that Amazon has introduced MV technology in its warehouses, where AI cameras and scanners watch the products stocked and automatically track which products go into which sorting streams.
Amazon’s software business Amazon Web Services (AWS) is identified by GlobalData as one of the main drivers behind machine vision and facial/video recognition. As CV requires a lot of computing power, particularly memory and processing capacity, running CV in the cloud has brought the technology to new customers.
AWS, Microsoft’s Azure and Google Cloud all offer platforms for training AIs both in the cloud and in local devices. GlobalData forecasts that as enterprises “increasingly embed AI-based software into technology and process development, CV as a Service (CVaaS) will become a crucial part of a business’s automation process.”
This all comes as part of the wave of AI disruption that is revolutionising business in the 2020s. GlobalData’s thematic research on artificial intelligence suggests that the market for AI platforms will reach $52bn in 2024, up from $29bn in 2019. Looking at present-day uses, it’s clear that a lot of that growth will be driven by computer vision.
Seen across sectors
Apple’s Face ID is probably the best-known application of computer vision through its face recognition properties. Identity management is also a major field of activity for banks, with face recognition adding an extra layer of security to their smartphone apps.
Other business applications of CV can be seen in the automotive sector. Honda, for example, has partnered with AI startup UVeye, which applies MV to vehicle inspections on production lines.
Seeing Machines meanwhile delivers driver-facing camera technology that detects signs of driver fatigue and distraction using computer vision. The company claims to have detected over 8.5 million distraction events, and works with major automotive clients like GM and Robert Bosch. More recently Tesla has confirmed it will rely solely on its vision-based cameras for self-driving functions, transitioning away from radar sensors.
CV has also infiltrated the supply chain leading to retail shelves. In the packaging sector, CV technology helps companies to reduce errors and waste during production. This is important as not only do faulty packaging and wasteful processes affect bottom line, but they also have significant consequences for the environment.
To get around this, Finnish fiber paperboard producer Metsä Board partnered with technology supplier Valmet to equip one of its mills with CV to improve the quality of paper it produces. The system uses MV to scan the paper produced and optimise paperboard surface quality in real-time with no human input. This system helps the company decrease its production waste, quickly grade changes, and provide consistent quality to the folding boxboard machine.
CV’s help in the fight against waste is also evident in the foodservice business. Hotel group Accor and retailer IKEA are among the earliest adopters of CV in their kitchens, cutting their food waste in half and saving over $880,000 annually. Online retailer Ocado meanwhile has invested £7m in automated chef Karakuri which can, among other things, optimise portioning in the kitchen with robotic precision.
Face recognition and fears
Having robots helping in the kitchen may require some time for human employees to get used to. But an even more startling development is the use of “seeing” robots monitor humans in the workplace, be it restaurant, warehouse or factory.
When it was announced pizza maker Domino’s was using computer vision to monitor quality control in antipodean stores, some outlets saw it as a harbinger of workplace surveillance deployed to keep employees in check. Another such deployment was revealed in a recent report from the Chinese Academy of Sciences on CV used on construction sites to monitor labourers. CCTV employing face recognition could determine whether employees were “loitering,” smoking or on their phones instead of working.
Facial recognition is arguably the Big Bad Wolf of AI. As GlobalData reports, the CV tech has been the subject of fierce debate in the last couple of years, with use in policing and surveillance under intense scrutiny.
“In the face of a regulatory vacuum,” its analysts add, “the lack of open public debate around facial recognition is compromising its legitimacy among the public and raising fears of mass surveillance.”
Privacy advocates like Ella Jakubowska have spoken to Verdict of their concerns. A policy and campaigns officer at the privacy advocacy group European Digital Rights, she stated that these technologies are “really infringing on every person’s right to privacy and data protection.
“But because they are being used in a way that amounts to mass surveillance they also have an impact across potentially the full range of people’s human rights.”
Such fears have seen Big Tech names like Amazon to publicly suspend sales of facial recognition tools to law enforcement. In theory, human rights concerns could halt the development of CV tech, or at least stall business investment. But in fact Amazon’s one-year moratorium from 2020 neither confirms or denies that it will stop selling tools to the federal government. The machine will resume operations soon, if it ever really stopped in the first place.
This doesn’t necessarily have to be bad news as long as everything is handled with good public engagement, according to Zak Doffman, CEO of internet of things-enabled security company Digital Barriers.
“Let’s be very clear: a computer system is significantly better at recognising an individual than any human being in the world is, and it can do it across many more people, but it’s not flawless,” Doffman told Verdict Magazine. “Therefore it needs to sit as part of a process to quickly work through and then eradicate mistakes.
“We feel that the industry has not helped itself by trying to run too fast and losing public support,” Doffman continues. “It needs to scale back. It needs to do this sensibly. We need to build on the success we’ve seen with identity assurance and educate the public that there is nothing to fear.”
By Verdict’s Giacomo Lee. Read the full feature on facial recognition in the latest issue of Verdict Magazine. Find the GlobalData Thematic Research: Computer Vision report here.