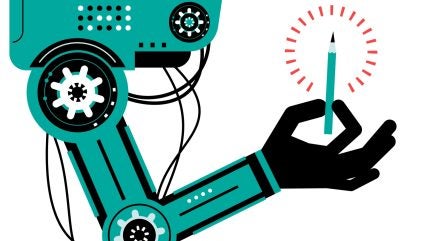
Artificial intelligence (AI) is said to be exponentially faster and effective at writing lengthy and complex clinical trial documents, but those using the technology see some limitations, which could mean a role for human medical writers endures.
Large language models (LLMs) can draft clinical documents in a fraction of the time their human counterparts need. Growing interest from the pharmaceutical industry to harness this technology has therefore made specialised writers worry their livelihood is under threat.
With LLM writing reaching a quality of around 80%–90%, human oversight can easily bring it up to 100%, says Chris Meier, managing director of the Boston Consulting Group. But the room for error in the pharmaceutical industry is slim, according to David Llorente, CEO of Narrativa, an AI-driven company supporting clinical work.
LLM writers show variable accuracy
LLMs are proficient in rapidly producing first drafts of study protocols, consent forms, clinical reports, and other trial documents, says Wing Lon Ng, director of AI engineering at IQVIA. He says: “The transformative potential of LLMs lies in dramatically improving efficiencies around initial drafting of clinical trial documents.”
Nevertheless, off-the-shelf LLMs such as ChatGPT cannot match the quality of professional medical writers, says Ng. Though LLMs can accurately employ terminology, they struggle with the clinical reasoning and logic behind documents and require human oversight to be employed effectively. Meier agrees with this. He and his colleagues tested the ability of OpenAI’s LLM GPT-4 to write key sections of clinical trial protocols and measured its accuracy by different metrics.
“It does a reasonable job in terms of terminology, in terms of getting some facts correct,” Meier says. The team found GPT-4 achieved 82% accuracy for the relevance and suitability of content and over 99% for appropriate use of terminology. But in clinical thinking and logic, scoring whether the LLM’s chosen endpoints and eligibility criteria agreed with guidance, GPT-4 achieved just 41.1% accuracy.
Off-the-shelf LLMs such as GPT-4 are trained on a limited dataset. Meier and his team, therefore, also tested a retrieval-augmented generation (RAG) LLM, wherein the AI was fed up-to-date information from sources that include ClinicalTrials.gov. This significantly improved writing quality. The RAG-LLM produced a clinical thinking and logic score of 79.9% while maintaining quality across other parameters and integrating contemporary references into drafts. Generally, RAG significantly enhances LLMs’ aptitude in drafting clinical documents, notes Ng.
Fundamental limitations constrain LLM medical writers
Current LLMs remain unable to take on the full role of medical writers in Llorente’s view. “The main problem with large language models is that they are actually language models, they are not knowledge models,” says Llorente.
Narrativa’s solution is to integrate broad LLMs with smaller statistical models and knowledge graphs trained to produce closer to 100% data accuracy, according to the company’s president Jennifer Bittinger. This limits the hallucinations—inaccurate or misleading content—often produced by LLMs.
Another challenge for LLMs comes from the shifting landscape of regulation governing clinical trial conduct. To further the up-to-date accuracy of LLMs, Ng advocates for regular feedback from LLMs to assess both accuracy and biases in generated text, ensuring patient data is representative of different ethnicities and genders, for example.
Patient data security remains a concern
Even if entirely accurate, there is concern surrounding the security of patient data collected by LLMs used for medical writing. The responsibility lies with major research and development (R&D) stakeholders—trial sponsors and contract research organisations (CROs)—to design strategies that continually update and work proactively against data breaches, says Ng.
Bittinger states Narritiva addresses security risks by housing its platform within private client cloud environments, ensuring the data never leaves private networks. However, cloud-based data storage can still prove vulnerable, as seen in January 2025 when it was revealed that DeepSeek, OpenAI’s Chinese competitor, suffered a data leak due to misconfigured cloud storage.
Therefore, Ng notes further measures to be taken, like rigorous anonymisation of data, strict control over data access, and regular audits. Encryption and multi-factor authentication are used to prevent unauthorised access, and knowledge graph-based filtering has proven effective in capturing harmful content produced by LLMs, says Ng.
LLMs to augment human capabilities, not replace them
What experts agree on is that LLMs do not mean the end for human medical writers. Ng states: “The most probable scenario is a hybrid approach where LLMs augment human capabilities, allowing experts to retain critical roles in oversight and complex decision-making and ensuring ethical and regulatory compliance.”
In Bittinger’s experience, medical writers themselves have begun to adopt this view. While several years ago, many professionals working on trial documents were against AI innovation in the field, she says now the industry largely sees the technology as a tool rather than a competitor.
“Because AI can help automate the low-hanging fruit … medical writers can then validate and do the quality control on those documents,” Bittinger states.
Meanwhile, AI developers continue to boost efficiency. At the 2024 Jefferies London Healthcare Conference, OpenAI account director Jovana Jordanova presented the company’s O1 reasoning model, demonstrating its ability to draft accurate and interconnected trial documents, applying human input in one document to all to maintain consistency.
But future innovation could encounter challenges. Speaking at the conference, Jordanova said it is essential to proactively foster AI adoption in the pharmaceutical industry to successfully deploy it in trial documentation, with collaboration between people and LLMs remaining central to the technology’s effective use.
According to Bittinger, there is also potential for legislative disagreement around LLMs in the clinical space, which may hamper adoption. Though she notes several pro-AI elements in the Trump administration such as the current head of its Department of Government Efficiency (DOGE) Elon Musk, she says: “There are quite a few folks who could be blockers [of LLMs] … it’s going to be a constant tug of war”.
Until uniform legislation on AI is reached, Ng warns that the currently varying regulations between or even within countries could render the use of LLM medical writers complex.
Regardless, pharma itself is steadfastly in favour of using AI from trial document drafting in Bittinger’s view. “This is not an option anymore, to use AI, it is a mandate, and it’s coming from the top down,” she states.